THE EQUATION • Issue no. I
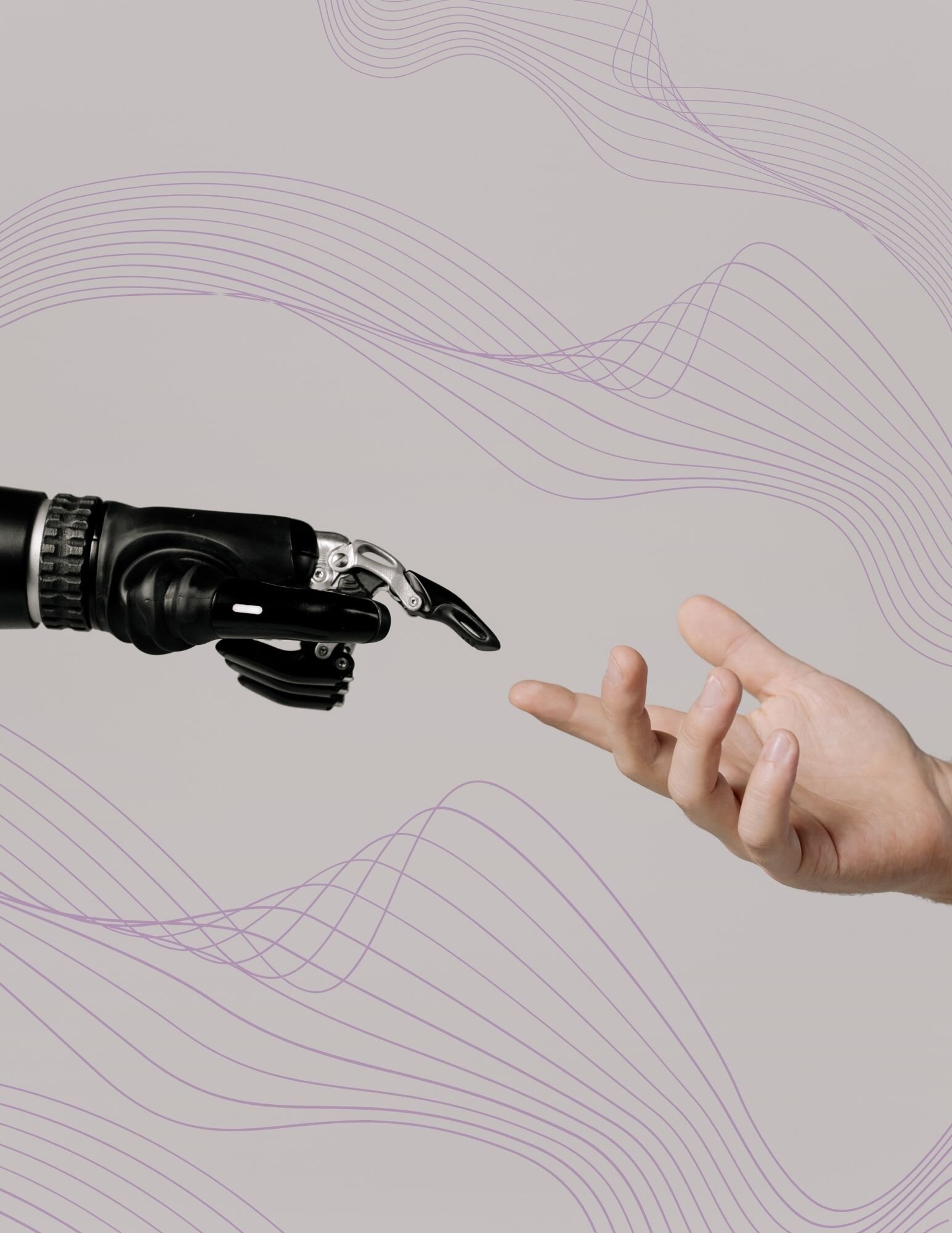
the equation • tech ethics quarterly • issue no. I
ETHICS AS A SERVICE
how the leaders of tomorrow’s technology are embracing ethics today
IN THIS ISSUE
Letter from the Editor • EI Team
What is Ethics as a Service • Helena Ward
Spotting Ethical Risk in AI & ML at Scale • Ben Roome
Unlocking Innovation with Ethics • Olivia Gambelin
The Origin of EaaS • Dr Anat Elhalal and Nathan Coulson
How to measure “good” AI • Charles Radclyffe
letter from the editor • the ei team
Dear Reader,
Welcome to the debut issue of the EQUATION, your tech ethics quarterly magazine.
Here at EI we have had the honor of working with companies who are leading the way into the new era of responsible tech by bravely committing to operationalising ethics in their technologies and workplaces. Because of this, we have seen first-hand the power of ethics in technological development, and now it is our mission to share these same insights with you.
Many months in the making, the Equation is a must read for anyone looking to lead in the tech industry. Packed full of curated studies, research, and best practices in tech ethics, this debut issue is the collaborative result of an incredible group of people who share the same aim: making tech ethics accessible and affordable for all.
Join us as we tackle the difficult questions surrounding technology head-on and seek to bring ethics to life through practical and elegantly simple solutions.
Happy reading,
The EI Team
what is ethics as a service? • helena ward
Allow me to introduce you to Ethics as a Service:
the technology game-changer you didn’t know existed until now.
Starting with the basics, ‘as a service’ is essentially the provision of something to a customer in the form of, literally, a service. In the context of the tech industry, this refers to the products, tools or technologies vendors provide over a network. Typically, we are accustomed to seeing software, platform, and infrastructure provided as a service. However, anything accessible at scale over network connection can qualify, music and mobility as a service being two great examples.
Now let’s bring ethics back into the equation. ‘Ethics as a Service’ does exactly what it says on the tin - provides ethical assistance, decision making, and advice as a service at scale. This is accomplished through contextually adaptable tools that aid in the ethical critical thinking necessary to successful design, development, and deployment of technology.
“EaaS incorporates ethics from the hearts & minds of designers and developers into work flows, and ultimately into the AI products released into society”
- Will Griffin, Chief Ethics Officer of Hypergiant
When you use Ethics as a Service (EaaS) you can expect to see two main outcomes: risk mitigation and innovation stimulation. On the one hand, ethics implementation reduces the harms that result from unexamined technological development. On the other, ethics unlocks new avenues of growth through value alignment. It’s a win-win kind of tool.
“It aims to make ‘unintended consequences’ a thing of the past, and to understand that stakeholders include everyone that your technology touches”
- Alice Thwaite, founder of Hattusia
With the power of Ethics as a Service in mind, it is important to recognize the importance of the human in utilising it - specifically, the trained professional human. Just as we look to data scientists for expertise in data management, lawyers for expertise in compliance, and accountants for expertise in taxes, so we should look to ethicists for expertise in ethics. An ethicist comes with a certain skill set, making them particularly adept at bringing EaaS to life within an organisation in order to detect and resolve the ethical bugs in our systems.
“All organizations need to stand accountable for how their use of data & AI is affecting people and society - ethical filters on AI applications are crucial to release the true power of AI”
- Anna Felländer, founder of AI Sustainability Centre
Ethics as a Service isn’t just a one and done box to tick. It’s an ongoing commitment to analysing and improving the impact of our technology, a commitment to continuously refining our decision making mechanisms, a commitment to bravely designing in accordance with our values.
Technology changed the way we live our lives forever, now it’s our turn to take back agency over our technology and change it for the betterment of our lives.
EI.
“We have witnessed far too many situations of what occurs when tech companies are either irresponsible, unethical, or uncritical as to the varied impact and potential misuses of its technology. Injecting a greater amount of ethical considerations in the process of how technology is developed and deployed is a recognition of the massive power that technology has in shaping our human condition and society at large, and the immense desire to ensure we are building a tech future aligned with the public interest."
- David Ryan Polgar, tech ethicist and founder of All Tech Is Human
The Responsible Tech Guide provides guidance to the diverse range of college students, grad students, young professionals, and career-changers that are looking to get involved in the growing Responsible Tech ecosystem. Often they are unsure about the careers, education, organizations, and pathways available for their future in the field. This guide is here to help.
spotting ethical risks in ai & ml at scale • ben roome
The key problem that most tech companies face with respect to aI & ML ethics
is not about being able to make the right decisions, but about making the right decisions in a way that is reliable and consistent across the entire organisation
Many companies fall into the trap of thinking “our team is made up of smart people who all have good intentions, and together we will avoid causing harm.” All the intelligence and good intentions in the world will come to nought unless there are clear and reliable processes in place to identify and mitigate risks that create transparency and accountability within the organization. In our experience, the most effective way to achieve scalable AI/ML ethics risk mitigation is to match the governance process with the technical practices of the company.
To succeed at AI/ML ethics, every company must develop the capacity to identify and mitigate risks reliably. Without this capacity, companies are likely to catch some but not all of the risks associated with their product and business model. Any unchecked risk can lead to serious harm for users and society. Companies we have worked with at Ethical Resolve
have gone about developing ethics capacity in many different ways. The main difference between the various approaches we have seen center on how ethics functions are distributed across organizations. Some companies choose to split up their AI/ML ethics function across multiple teams, while others take a more centralized approach. While there are many possible organizational approaches, the key to successful AI/ML ethics practices is to ensure that people with the relevant expertise have thought carefully about the impacts of a product or feature before it is released and makes contact with users. Doing this right once or twice in an ad hoc fashion is comparatively straightforward; doing it consistently across the organization for every product or feature update is much more difficult. In our experience at Ethical Resolve, it is best achieved by matching governance practices to the infrastructure stack. To reflect this, our process of risk spotting deploys a governance model that mirrors the technical stack on which software products are built.
Ensuring that the right people have thought carefully about potential negative impacts requires a review process.
Some companies include ethics review as part of their Product Requirements Documents (PRD), while others include product review as a component of the launch calendar. Independent of the format, once an organization has agreed to review its products for ethical impact before launch, the problem becomes one of logistics. How can the review team (which usually comprises members from product, engineering, trust & safety, legal, responsible innovation, and/or AI/ML ethics teams) ensure that all potential risks of the product have been identified and properly addressed before the product is launched or updated? How do the disparate teams implicated in review processes efficiently achieve cross-functional visibility into the datasets and product decisions and tradeoffs?
When we look into AI/ML products for risk, we see it emerging in three key areas: the data sets, the model(s) generated from the data (including any algorithms used to generate that model), and the product design itself. Regardless of what programming language or software a company uses to create them, data sets, models and products each represent a section of the company’s technical stack. Whether the model is built using machine learning or not, it is designed to provide information about a past or present state or make predictions about a future state. Using data to make decisions is about focusing on key pieces of a dataset in order to tell us something about the world. The way the dataset is constrained to focus on some specific aspect of the data will have impacts on decisions that are made about the reality the dataset represents.
When data scientists design a model in order to generate predictions or decisions about reality, we have to think carefully about the assumptions that are built into that model, which usually begin in the dataset. If we want to understand the impact of a product, we need to be very clear about all the people, both users and nonusers, who might be impacted by that product. All these assumptions and potential impacts constitute important contextual information that is often not included by the teams who have built the model and/or product.
This contextual information is critically necessary to conduct a successful review.
However, machine learning development is essentially a process of stripping context in order to create a computationally efficient model. Every step down the machine learning development stack—from data lake to dataset to preliminary model to deployment model—is a refinement away from the original context of data collection, all with the purpose of creating a predictive model that will then be deployed back into the context-rich real world. Thus, when companies attempt to conduct reviews at scale, review teams often have to spend time searching for the relevant contextual information by contacting the team directly and inviting them to a meeting. Every party involved with the review process requires different insights into the development process, creating a backlog of demands on the product team. This is not the most efficient solution, and it certainly won't scale across a large organization.
For this reason, reliable governance practices that track the relevant contextual information about the product need to be systematically emplaced in order to provide the review team with what they need to determine if the product is likely to have negative impacts on users and society. We have taken to calling this a “governance stack,” which is essentially the retention of ethically-relevant “metadata” throughout the development process.
Think of a governance stack as a countervailing force against the loss of context needed to make sound ethical decisions.
© Ethical Resolve
What do these governance practices look like?
Excellent examples of successful governance techniques are customized versions of “Datasheets for Data Sets” and “Model Cards for Model Reporting” developed by Timnit Gebru and Margaret Mitchell et al. When a data scientist uses a dataset to create a model that is going to be deployed to a product, they must support the review process by providing contextual information about the provenance of the dataset and the purpose of the model they are creating. Similarly, when a product team is ready to deploy their product, they need to provide relevant contextual information to the review team in a systematic way in advance of the review.
Key questions about datasets include:
By what specific means was this data collected?
Was the data collected with the consent of the data subjects?
Does this dataset include third-party data?
Does the dataset contain any personally identifiable information or proxies for PII?
Does the data include sensitive demographic data, or can individuals’ sensitive demographic status be inferred from the dataset?
What types of regulated data are included or may be inferred?? (e.g., medical, financial, etc.)?
Does this data reflect the composition of the populations about which the deployed model will be making predictions or decisions?
Key questions about models include:
What is this model meant to predict or make decisions about?
Can this model result in negative disparate impact in any of the following areas?
Financial
Social
Physical
Psychological
Model Details. Basic information about the model.
Person or organization developing model
Model date
Model version
Model type
Information about training algorithms, parameters, fairness constraints or other applied approaches, and features
Paper or other resource for more information
Key questions about products include:
What is this product intended to do?
How might it result in negative or disparate impact to users or society in any of the following areas?
Financial
Social
Physical
Psychological
How might it differentially affect people who are in a disadvantaged social or economic category?
Once these and similar questions have been answered by the relevant team, members of that team can be invited to take part in the review and think creatively to address any issues that may have surfaced.
In effect, these questions ask the data scientists and product team members to engage in risk spotting before those products are sent to review. Rather than asking the team to simply complete a checklist, this approach to governance invites the team to think creatively about how risks may be addressed in the product.
We recommend that all companies deploying the practices described above provide the relevant training to their teams about the importance and successful deployment of these practices. Teams that offer a perfunctory response to risk spotting and mitigation worksheets may need to be provided with further training in such practices. Organizations that do not properly socialize these activities can face pushback
from teams that voice concerns about onerous reporting practices stifling innovation. It is critically important for companies to right-size their governance practices so that they are carried out in good faith by the people whose task it is to complete them. These practices do not have to be problematically time consuming, can be conducted the first time with another team member who has expertise in this area, and can be approached as a generative thinking activity rather than a critical one.
These processes are ultimately about accountability and transparency. A commitment to internal transparency results in greater organizational efficiency to identify risks. This in turn translates to ease of regulatory accountability, which puts the organization in a better position to respond to and guide regulatory practices as they emerge. The organization needs to see what is happening as its products are developed and ensure that product and data science teams do their part to de-risk the things they build before they cause negative impacts.
Using these types of governance practices, any organization can more easily provide the relevant information to the product review team in order to help them make decisions effectively. Some models or products might be flagged for closer review based on an obvious risk to users or society more broadly, while others that are recognized to have low risk can be passed after a much more limited review process. The point of effective governance practices is to create a system by which risk spotting and mitigation happens at the phases of product and model development where negative impacts can be avoided.
Once the review team has the relevant resources it needs to make a go/no go decision about a product, the process of conducting review at scale becomes far more tractable. Companies are able to spend more time reviewing potentially risky products and making changes to them to address those impacts. When products that are at lower risk for negative impact can be passed through the review process with less friction, this helps to avoid spending unnecessary resources where they are not needed. As risk identification is deployed such that governance techniques mirror the technical processes of the company, the company develops the capacity to make the right decisions at scale.
EI.
Ethical AI Health Check
The Ethical AI Health Check is a quick, but critical, first screening to discover an organization’s opportunities, potential pitfalls, and ways to release the real power of AI. That is, ethical and sustainable AI for innovation humans can trust.
Quantifying Ethical Impact
Our objective is to quantify the impact of operationalizing ethics in the AI development process. We believe that quantifying the outcomes of operationalizing ethics in AI development is a necessary first step in the widespread adoption of ethical frameworks and guidelines.
unlocking innovation through ethics • olivia gambelin
When someone claims ethics is a blocker to innovation,
I tell them it’s a shame that they are discounting one of the most powerful innovative tools we humans have before even giving it a try.
Due to the initial success of the Silicon Valley startup, innovation has become something we associate with the ever accelerating “fail fast fail often” iteration cycles. Speed, optimisation, productivity - these somehow seem to have become the indicators for the innovative in our modern tech world. However, despite what investor dollars may lead us to believe, moving fast and breaking things is actually not what makes for strong innovation, let alone an accurate definition.
Innovation, much like ethics, is a term we are all accustomed to hearing yet can only give a vague definition for if ever asked. So let me briefly provide one - simply put, in the context of business, innovation is the introduction of a new idea, method, or device that benefits the company. A surprisingly simple definition without even an honorable mention to efficiency or speed, we begin to see that just maybe
there might be more to this whole innovation thing than our silicon startup standard has led us to believe.
In the spirit of introducing a new beneficial concept, allow me now to bring ethics back into the conversation. Because ethics requires time and effort, it has, until now, been misclassified as an innovation blocker. However, as we are seeing, this is not actually in conflict with our understanding of true innovation. In fact, I have seen quite the opposite, finding that the startups and enterprises who take the time and effort to consider their values and examine how to use these values as critical decision-making factors at scale, are really the ones pulling ahead to lead this next era of technology.
Why is that?
First, consider where successful innovation begins. When we need to come up with the next new best thing, where do we start? Is it with a sudden spark of creativity, a change in critical perspective, a breakthrough in cutting edge research? As any student of innovation can attest, there is no single path to success, as methods and best practices come and go out of fashion often quicker than they can be defined. However, all effective innovations do have one single thing in common; they all start with a problem that needs solving.
It is safe to say technology has mastered the ‘wow factor,’ as we are never short of cool gadgets and fancy machines that make magic look like child’s play. However impressive these are, new and shiny wow factors do not necessarily make for successful innovations. If the new thing, be it a feature, product, or service, is not created to solve a specific problem felt by your targeted user base, then it will only ever remain a cool idea gathering dust on the shelf. Successful customer-centric innovation doesn’t come from turning out creative idea after creative idea, but rather aggressively seeking out the problems yet to be solved.
Seek new problems, not new solutions. Elegantly simple, yet what does this have to do with the millennia old study of ethics?
Ethics, when used as a conceptual tool, can help in both identifying and defining your newest problem to solve. Even in the heart of the Silicon Valley, true technology innovation is declining to be replaced by feature iteration as there are only so many ways dating apps, HR hiring tools, cloud storage, etc. can be reinvented. Although technology may continue to push some boundaries, AR sunglasses and pizza delivery robots will only ever be wow factors, they will never be solutions to human needs.
There are however, very real and urgent needs when it comes to the ethical design and use of technology. For example, we as users need agency over our own data, fairness in algorithmic decision-making, and accountability for the impact of our tech. But that’s not all. Expanding out of the narrow context of technology, we as human beings need to improve our
wellbeing to find long-term happiness and fulfillment, we need to develop better habits and processes for taking care of our planet, we need to look for ways to connect with each other rather than divide ourselves. All of these needs, in one way or another, point back to what we value in life. And ethics is simply the conceptual tool that allows for us to understand what it is we truly value and how to align our actions to achieving those values.
It is this sweet spot between our values and actions that ethics unlocks a whole new world of potential true innovative solutions to real human problems. Our favorite ancient Greek philosophers defined ethics as the tool that aids in the pursuit of the good life worth living. Shouldn’t we also be able to use ethics in the pursuit of the good technology worth developing?
But wait, that’s not all. Ethics enables us to fine-tune the problems worth investing time and resources into innovatively solving, but it also empowers the informed and strategic allocation of such efforts.
By definition, innovation is new territory, and new territory is inherently risky. There is no guarantee that things will go as planned, let alone how much the final outcome will resemble the original idea. Because of this inherent risk, one of the anthems of innovation has become to fail fast and fail often. The quicker you can test the validity of an idea, the quicker you can arrive at the one that will stick, and all the failures you meet in between should be embraced as learning moments for improvement.
Although overcoming the fear of failure is important for successful innovation, there still remains select cases in which failure is just not an option. When your user’s well being is jeopardised, that is not just
another cost of innovating in the cycle of fail, learn, repeat. That is a line that you should be highly cognisant of at all times and taking active measures to ensure is not crossed.
It is also where ethics again plays a strategic and crucial role in the innovation process. Understanding the difference between instances in which failure is advantageous versus hazardous is a matter of understanding the ethical limitations of a project. Everytime that thin line is crossed, you are acquiring ethical debt, which if goes unchecked for too long will inevitably end in disaster. By instead utilising ethics to fully realise the limitations of your desired innovation, you are establishing the guiding constraints that will enable you to focus your efforts with confidence. In other words, ethics ensures that the risks you are taking are solely strategic business decisions and not decisions that compromise the well being of your end-users, indirect stakeholders, or even society at large.
With all this in mind, let us return to the original misconception of ethics being a blocker to innovation, as it is clear now that it was not actually ethics being misunderstood but in fact innovation itself.
True innovation does not start with a creative spark of an idea, it starts with a deep understanding of a problem in need of solving. It is also not contingent on failing fast and often, instead it depends on thoughtful reflection and strategic risk-taking. Taking this refined understanding of innovation to heart, we are able to see the game-changing innovation tool ethics can be when it is utilised to identify and clarify the problems worth solving and to enable through calculated risk-taking.
Innovation plus ethics equals long-term solutions built for human problems, now isn’t that a lovely simple equation?
EI.
the origin of EaaS • interview with dr. anat elhalal & nathan coulson
This debut issue of the Equation focuses on defining and exploring Ethics as a Service,
but where did the term first originate?
A collaborative effort between authors from Digital Catapult and Oxford Internet Institute led by Jessica Morley, the paper “Ethics as a Service: a pragmatic operationalisation of AI Ethics” was released in February 2021 coining the term. Critically examining the current efforts in AI Ethics, this piece explains the gaps in our efforts and offers EaaS as the much needed solution to bringing ethical values into practical technological action.
We had the honor of sitting down with two of the authors, Dr. Anat Elhalal and Nathan Coulson from Digital Catapult, to discuss the research and thought that inspired the birth of Ethics as a Service.
Digital Catapult Ethics Programme won the CogX "Outstanding Achievement in the field of AI ethics" award a the Machine Intelligence Garage won the "Outstanding AI Accelerator"
Ethical Intelligence: What was your role with Digital Catapult during the time you helped author the piece “Ethics as a service: a pragmatic operationalisation of AI Ethics”?
Dr. Anat Elhalal: At the time I was the Head of AI/ML Technology at Digital Catapult, accelerating companies on their ML journeys with emphasis on responsible innovation. Providing technological leadership across the programme, I focused on identifying innovation and adoption barriers specific to Machine Learning and AI, along with developing the interventions to address them.
Nathan Coulson: My role was as a technologist leading on the technical and ethics aspects of our startup acceleration programme, the Machine Intelligence Garage. The work undertaken within the Machine Intelligence Garage by the Ethics Committee Advisory Group and the MI Garage team informed the theoretical formulation of “Ethics as a Service”. Through 80+ ethics consultations (1 hour structured and facilitated meetings between a startup and two members of the Ethics Advisory Group) we grew our practical knowledge of applied ethics.
EI: What first inspired the term ‘Ethics as a Service’?
AE: In 2017 we launched the Machine Intelligence Garage: Digital Catapult acceleration programme for Machine Intelligence startups. I started promoting the idea of an independent Ethics committee as part of the programme right from its inception. I found a great mentor and partner for my aspirations in Prof Luciano Floridi from Oxford’s Digital Ethics Lab, who took the role of Chair of the Ethics committee. Together with my team, we recruited a high profile steering board as well as an advisory group for the Ethics committee, and started building an ethics service from the ground up, based on first principles and our experience working with companies.
We created and published an AI Ethics framework, designed a consultation service for companies, and started an industry working group. We also invested in creating a typology of AI Ethics tools (published here), which was our first collaboration with Jess Morley from the Digital Ethics lab. Together, we continued to define the theoretical foundations of our practical work in AI Ethics. The “Ethics as a service” term was coined by Jess as part of this process, although no one was sure how come we didn’t think of it earlier!
EI: In what ways was the piece a reflection of the work you were doing with Digital Catapult?
NC: The piece was informed by the real experience of providing an ethics service to startups although the academic foundations and theoretical contributions were provided primarily by the lead researcher Jess Morley with input and guidance from the other authors (Anat and Frankie from DC and Luciano from OII).
EI: What is the biggest blocker to widespread use of Ethics as a Service?
NC: Sustaining the cultural change necessary to place ethics as a co-equal aspect of the product development process (along with for example UX, Product Management, agile AI and software development processes).
AE: At this day and age, investing the time and effort in responsible AI innovation slows product development processes down, and normally adds friction. I strongly believe that the long term benefits of responsible AI innovation outweigh the short term costs. However more data is needed to convince most companies to invest their precious resources in AI Ethics.
EI: What is the biggest benefit a company can gain from using Ethics as a Service?
AE: Robust future proof products and services that are more appealing to investors, clients, employees, and society.
NC: Improving their products and services through proactive and pre-regulation risk mitigation while unlocking further benefits like increased user trust, employee retention and investor buy-in through ethical alignment.
EI: As the Tech Ethics industry develops and new best practices will emerge, do you feel that Ethics as a Service is here to stay?
AE: We are pioneers in this field, learning from our mistakes as we go. I hope to see new improved ideas emerge as a result.
NC: Yes, no doubt there will be new developments and refinements but we have seen some evidence in the real world that an “ethics as a service” approach is valuable.
EI.
CASE STUDY
A collaboration between Loomi, an artificial intelligence (AI) startup, and Digital Catapult and its Ethics Committee, this deep-dive highlights the value that responsible approaches offer businesses developing AI products and demonstrates how long term commitment to ethical processes or methodologies can help AI companies to achieve positive commercial outcomes.
Challenges to Responsible AI Adoption
In January 2020, Digital Catapult convened the Industry Working Group, with its members assembled from UK-based organisations actively engaged in AI deployment and procurement. The objective was to define what a working group of industry peers can do to advance best practices and responsible AI adoption.
how to measure “good” ai • charles radclyffe
The problem with discussing Ethics & AI
is that we’re talking about two terms which have the same rather frustrating quality: they both mean different things to different people.
Take the example of AI. What is one team’s triumphant implementation of AI technology, is, to another group of technologists, derided as being mere robotic process automation. Even within the field of machine learning there are techniques which are seen as mere statistical analytics, and so the boundary of what is ‘true-AI’ (and I’m not talking about super-intelligence) is continuously morphing and shifting – a problem exacerbated by over-zealous marketeers and ill-informed journalists.
As for the challenge with Ethics; it is, by definition, a field of enquiry where what is ‘ethical’ is simply different to each of us. What’s OK for me might well make you recoil. That’s OK – we’re both being ‘ethical’ in that we are each able to make ethical evaluations of our and others actions. Except psychopaths, of course, and there might even be a few of those in the tech industry…
The issue at hand though is what do companies need to do in response to growing pressure around AI ethics and digital ethics more broadly?
Digital Ethics is a term synonymous with Digital Responsibility – essentially the body of activity that means that a company is acting appropriately with its digital technology aligned to goals driving environmental sustainability, greater social justice. The term ‘acting appropriately’ more accurately refers to whether an organisation has the necessary corporate governance in place so that the commercial goals and risk appetite set by the CEO and governed by the Board is delivered by those on the front-line developing or marketing such technological systems.
This is where the term “ESG” comes from. The acronym refers to the extent to which an organisation lives values of environmental sustainability and social justice through its corporate governance. Some ESG assessments are made based on corporate filings and other formal disclosures, others are inferred from social media and newswire sentiment, still others come from the companies themselves via informal disclosures through surveys and the like. Whatever the method, the goal is to evaluate the ESG risk that an organisation has and understand whether its strategy is likely sufficient to mitigate such risks in the short, medium, and long term.
It’s clear that most of the debate around AI ethics is focused on social justice questions and blind to other factors such as the environmental sustainability of a data strategy or its computational infrastructure. The risk of such a narrow approach is that there is a high chance that the implementation of AI ethics best-practice is disconnected from ESG strategy and that the organisation may well be blind-sided to risks that it had not considered, or focused on those which stakeholders are not actually the most concerned about.
The best organisations seek to integrate AI ethics within the context of ESG, and thereby ensure that the organisational priorities are lived through the technology and the appropriate guardrails are communicated to the market through the right channels, and performance metrics of technology systems are evaluated and eventually published alongside other ESG measures such as carbon footprint.
To not act now is to compound the risk. The European Commission recently published draft rules on governing AI which paved the way for a regime of disclosure to be instigated that will normalise the external reporting of factors of governance in a way that might seem foreign currently to those on the front-line. With the spectre of such regulation, there is an imperative for Chief Digital Officers or the most senior accountable executive for AI and autonomous systems to consider the implications of their innovation in the context of ESG.
EI.
If you’re interested in learning more about this topic, and would like to compare the ESG scores of nearly 300 of the world’s largest organisations with respect to the quality of their digital governance, then visit www.ethicsgrade.io or get in touch with me via Linkedin.
The Data Oath
At The Data Oath, we take the position of common sense and rational expectation when designing frameworks for ethical data.
Metaphors, data and UK Policy
Hattusia partnered with Defend Digital Me and The Warren Youth Project to consider how the metaphors we attach to data impacts UK policy.
thank you to our contributors
-
Will Griffin
Will Griffin is Chief Ethics Officer of Hypergiant, an enterprise AI company based in Austin, Texas. He received the 2020 IEEE Award for Distinguished Ethical Practices and created Hypergiant’s Top of Mind Ethics (TOME) framework, which won the Communitas Award for Excellence in AI Ethics.
-
Anat Elhalal
Machine learning leader with over 15 years’ academic and industry experience; accelerating companies on their ML journeys with emphasis on responsible innovation.
-
David Ryan Polgar
David Ryan Polgar is the founder & director of the non-profit All Tech Is Human, which is committed to building the Responsible Tech pipeline.
-
Alice Thwaite
Alice Thwaite is a technology philosopher and ethicist who specialises in creating democratic information environments. She is the founder of Hattusia, a technology ethics consultancy, and the Echo Chamber Club, a philosophical institute dedicated to understanding what makes information environments democratic.
-
Anna Felländer
Anna Felländer is the founder of AI Sustainability Center offering an ethical AI governance platform.
-
Nathan Coulson
Nathan Coulson is a Senior Technologist for Responsible and Ethical AI at Digital Catapult, previously worked for multiple tech startups and accelerator programmes and has a mixed academic background including both social sciences and AI.
-
Charles Radclyffe
Charles is a serial entrepreneur who has focused his career on solving tough technology challenges for some of the world's largest organisations.
-
Ben Roome
Ben Roome, PhD is a data ethicist, epistemologist and education technology entrepreneur working toward a future where all living beings can flourish.
further learning
Ethics as a Service
DISCOVER WHAT EAAS CAN DO FOR YOU
Take our quick ethics journey survey to receive a custom ethics strategy today.
-
RT @_jessiejsmith_: I recently helped develop and deliver a 2-lecture series on Data Ethics with @ethicalai_co! If you're interested in… https://t.co/0Ejz595P43
-
Did you really think we wouldn't use generative AI to create the cover image for our #generativeai themed magazine… https://t.co/oEsJLVjve5
-
Check out @4TUethics's blog series focusing on the people putting ethics into action, including our Founder & CEO… https://t.co/xTkGDJV9X0
Thank you for reading. Be sure to subscribe for future issues delivered straight to your inbox.
© 2021 Ethical Intelligence Associates, Limited